A large PARSEC team published a paper in 2022, in which they aimed to help researchers understand the challenges of reproducing Deep Learning (DL) publications, mitigate reproducibility gaps, to make their own work more reproducible. They built on the work of three other teams and added recommendations organized by (a) the quality of the data set (and associated metadata), (b) the DL methodology, (c) the implementation methodology, and the infrastructure used. To our knowledge, this is the first initiative of its kind to address the problem of reproducibility in remote sensing imagery and DL problems for real-world tasks. We hope this paper lowers the barrier to entry for the DL community to improve research. Following the lifecycle mantra: reproduce! then replicate! With the goal of improving reproducibility!
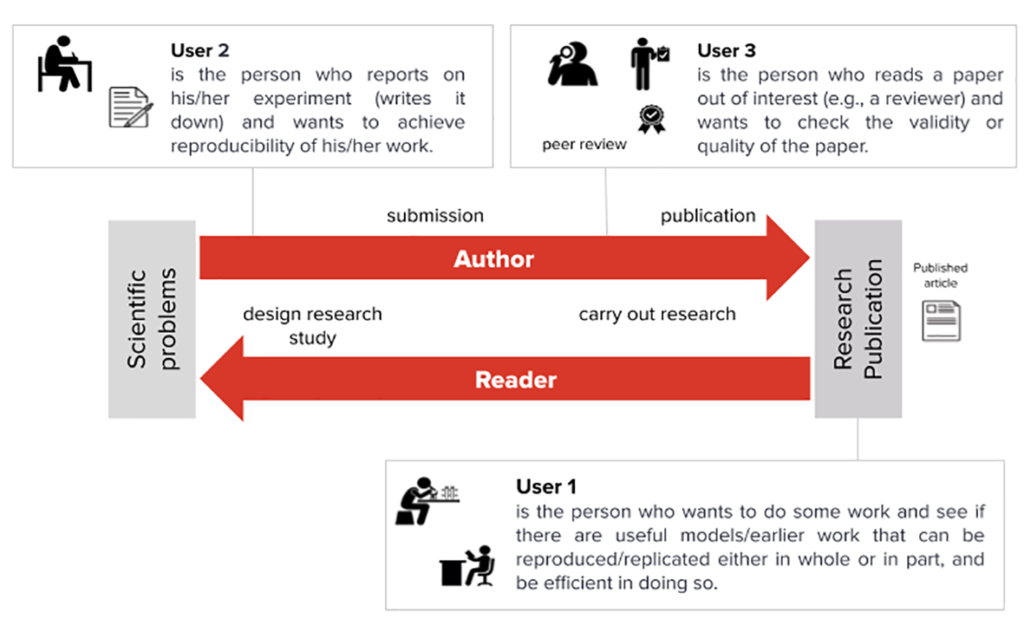
citation: Machicao, J., A. Ben Abbes, L. Meneguzzi, P. L. P. Corrêa, A. Specht, R. David, G. Subsol, D. Vellenich, R. Devillers, S. Stall, N. Mouquet, M. Chaumont, L. Berti‐Equille, and D. Mouillot. 2022. Mitigation Strategies to Improve Reproducibility of Poverty Estimations From Remote Sensing Images Using Deep Learning. Earth and Space Science 9. Doi: 10.1029/2022EA002379